About Hugging Face
Hugging Face Overview
Hugging Face is a collaborative platform designed for the machine learning community to build, share, and enhance models, datasets, and applications. It serves as a central hub for developers, researchers, and organizations to explore cutting-edge AI technologies, aiming to facilitate innovation in artificial intelligence through open-source collaboration.
Hugging Face Highlights
- Access to over 400,000 models and 100,000 datasets for diverse AI applications.
- Support for various modalities including text, image, video, audio, and 3D data.
- Enterprise solutions offering advanced security, access controls, and dedicated support for organizations.
- Robust open-source libraries like Transformers and Diffusers for state-of-the-art machine learning development.
FAQ
Q: What are the main use cases for Hugging Face?
A: Hugging Face is primarily used for building and fine-tuning machine learning models, accessing datasets for training and evaluation, and deploying applications for various AI tasks including natural language processing and image generation.
Q: How much does Hugging Face cost?
A: Hugging Face offers a pricing model starting at $0.60/hour for GPU compute and $20/user/month for enterprise solutions, with various tiers depending on the features and support needed.
Q: What technical requirements or prerequisites are needed to use Hugging Face?
A: No specific requirements were mentioned in the source, but users typically need a basic understanding of machine learning concepts and access to a compatible programming environment.
Q: How does Hugging Face compare to similar tools?
A: Hugging Face stands out due to its extensive library of pre-trained models, strong community support, and a focus on open-source collaboration, making it a go-to platform for both individual developers and large organizations in the AI space.
Q: What are the limitations or potential drawbacks of Hugging Face?
A: No specific limitations were mentioned in the source, but users may encounter challenges related to model performance or compatibility, depending on their specific use cases and technical expertise.
More Generative Art Tools
BlueWillow
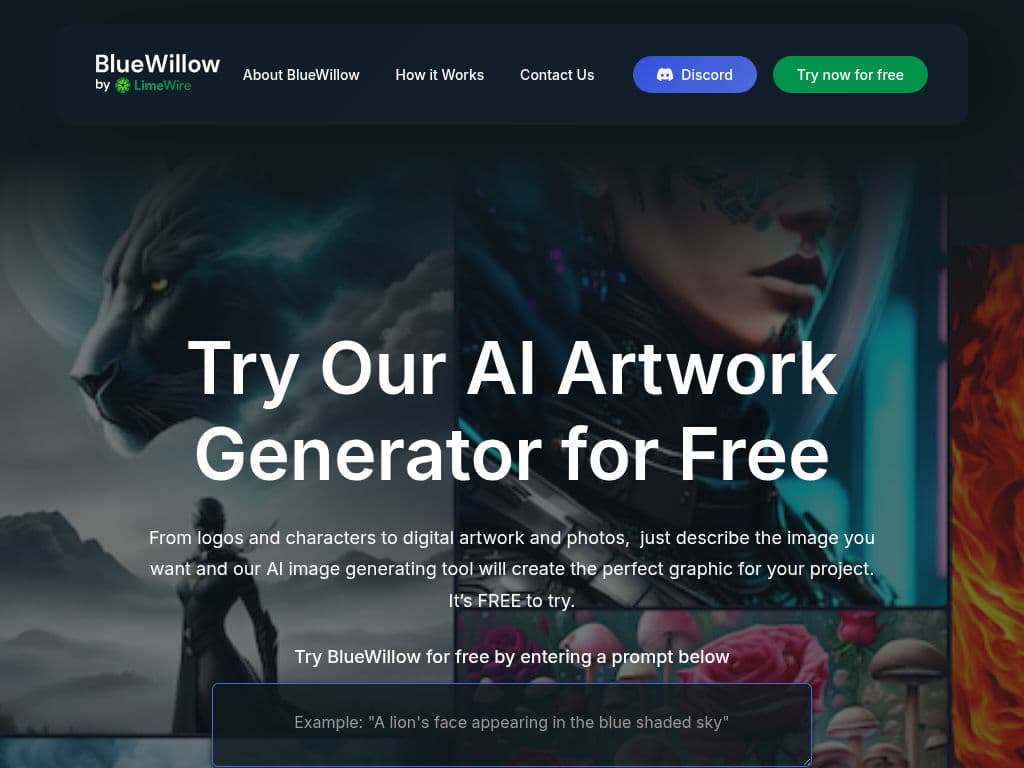
BlueWillow Overview BlueWillow is an AI-powered artwork generator that transforms user prompts into stunning graphics, including logos, characters, an...
Midjourney
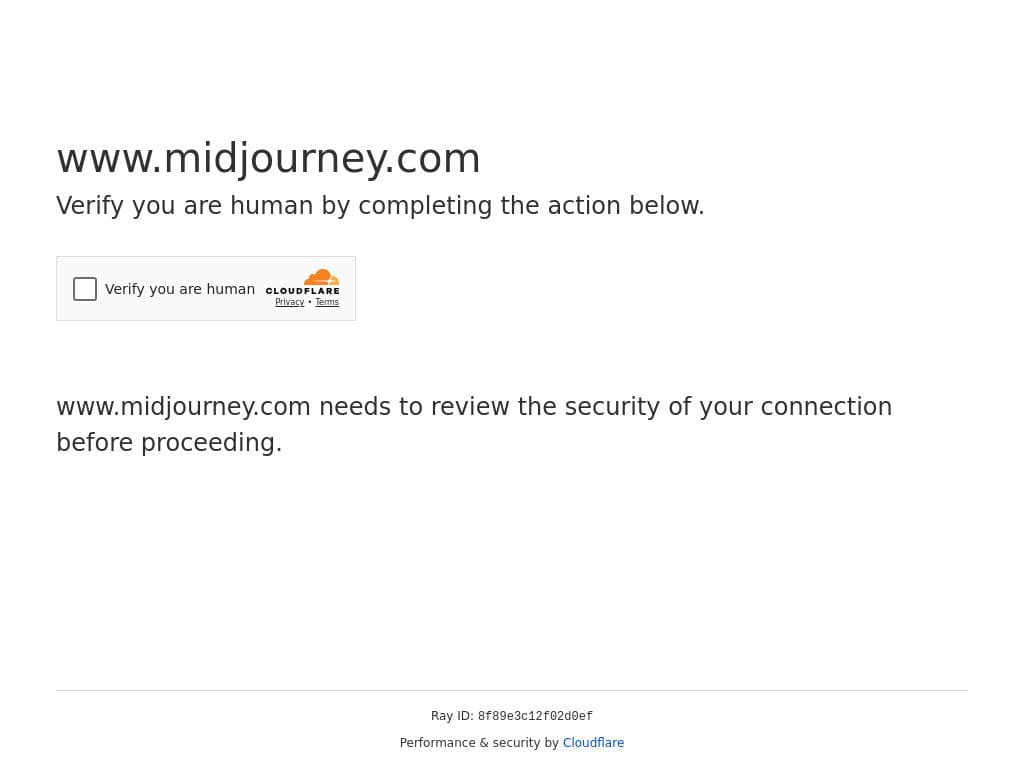
Midjourney Overview Midjourney is an advanced AI-powered tool designed for generating high-quality images from textual descriptions. Aimed primarily a...
Maze
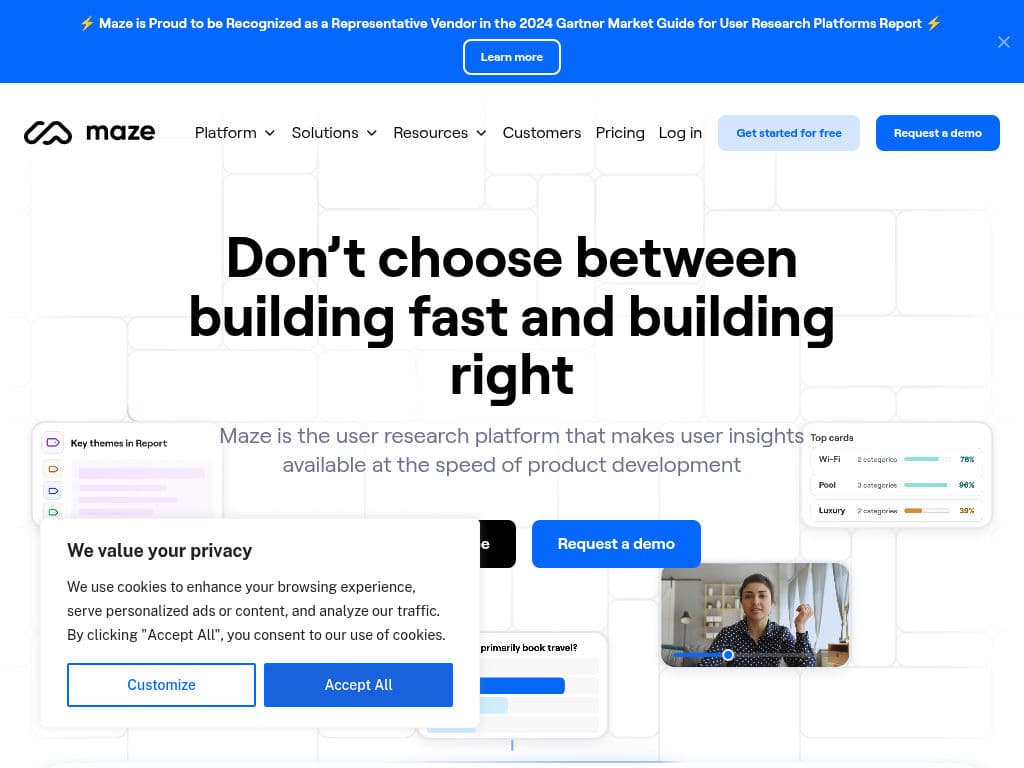
Maze Overview Maze is a user research platform designed to accelerate the collection and analysis of user insights throughout the product development ...